Stock markets are facing a major challenge: Algorithms
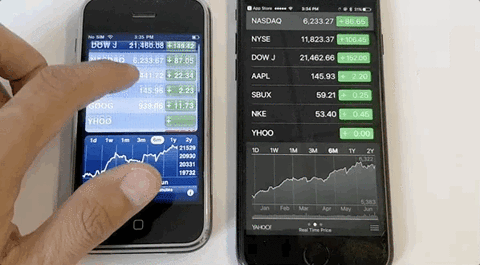
(Illustration: Giphy)
Algorithms have a hold on the stock markets that has fuelled the need for regulation. But how do we regulate what we don’t understand? The second generation of trading algorithms are designing their own investment strategies – and they are so complicated that we are unable to understand them.
ResearcherZone | 12. Sep 2022
By Christian Borch, former Professor at the Department of Management, Politics and Philosophy, Copenhagen Business School, now Professor at the Department of Sociology, University of Copenhagen
In 1995, Barings, a British merchant bank, collapsed because Nick Leeson – a trader working in the bank’s Singapore office – had been speculating in shares for years without the bank’s approval.
Although Leeson’s investments initially paid off handsomely, over time, they accumulated considerable losses, which Leeson attempted to cover with increasingly risky transactions.
When his house of cards finally hit the deck, Leeson pulled the over 200-year-old bank down with him.
Leeson received a prison sentence and subsequently published a book, Rogue Trader, recounting the experience, which was later turned into a film with the same name.
Leeson’s actions could prompt psychological musings about what fuels speculative behaviour in the financial markets and risk-related behaviour more generally (in fact, Leeson studied psychology after his release).
Own versus corporate interests
Leeson’s conduct also illustrates what social theorists refer to as principal-agent problems, which concern situations where a person or group of people (the principal) delegates decision-making competence to another party (the agent).
The problem arises when the agent has an incentive to act in a way that prioritises their own interests above those of the principal.
In Leeson’s case, he was employed to administer the bank’s money through investments, but he did so in a way that exceeded his authority, in the hope of obtaining personal bonuses.
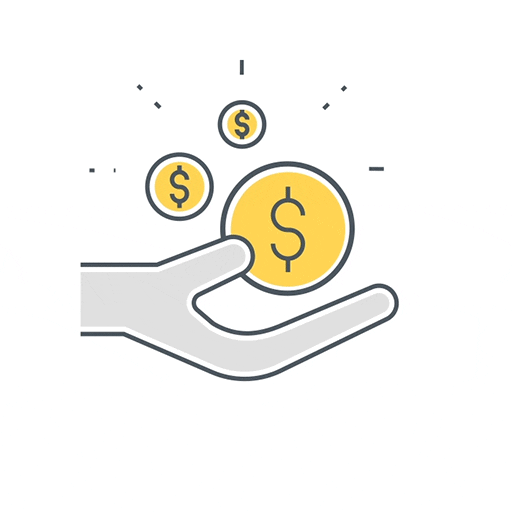
The agent failed to act in the principal’s interests, and that ended up costing them both dearly.
I chose to begin with a case that is almost 30 years old because it neatly illustrates the fundamental problem between agents and principals.
Since Leeson’s downfall, computer algorithms have begun gaining ground and dominating the financial markets, and so it is no longer clear whether or not the agent is a person.
Before I discuss the implications of this, it is relevant to dive a little deeper into classic principal-agent debates.
How do you get an agent to act in the principal’s best interests?
Principal-agent problems arise in many kinds of contexts, but few are as spectacular as the Leeson example.
Originally, economists Michael Jensen and William Meckling formulated the principal-agent problem as a description of the relationship between company shareholders and the management.
The management can be said to act on the shareholders’ behalf, but the latter has no guarantee that the former are not putting their own interests first.
Over the years, researchers have proposed various solutions for managing the basic problem of potential conflicts of interest when delegating decision-making competence, so that the principal’s and agent’s behaviour align.
For example, it has been suggested that the agent’s behaviour can be aligned with the principal’s by introducing special financial incentives or clear contractual frameworks.
Another suggestion highlights that, to the extent that the agent’s tasks can be clearly and precisely specified, the principal can more easily monitor any deviations from the agent’s authority and instructions.
From humans to all-dominating algorithms
At their core, social theory discussions of and potential solutions to the principal-agent problem both revolve around the agent being seen as a person:
The principal-agent problem arises because the interests of a physical individual (or group of individuals) can conflict with those of the principal.
This perspective neatly encompasses the Barings bank example. By speculating beyond his mandate, Leeson could obtain considerable personal bonuses, but at significant risk to the bank.
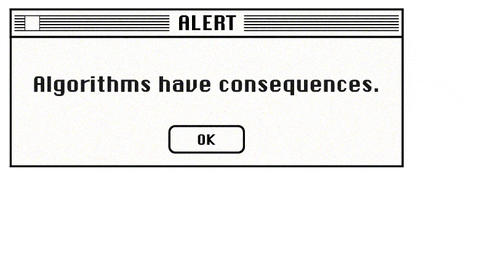
Banks worldwide still employ large groups of individuals to invest in financial products. However, in recent decades, the financial markets have undergone a transformation that reveals the principal-agent problem in a new light.
Rather than people made of flesh and blood, now primarily fully automated algorithms are sending buy or sell orders to stock exchanges.
Calculating the scope of these algorithms is difficult, but estimates indicate that fully automated algorithms in some markets – for example some stock markets – are responsible for 99% of the total volume of orders.
How first-generation algorithms work
Financial algorithmic trading can be divided into two generations, since first-generation algorithms follow strategies defined by humans, whereas second-generation algorithms develop their own strategies.
The first generation of fully automated algorithms took off in earnest at the beginning of the 21st century. The hallmark of first-generation algorithmic decision-making is that humans design it from end to end.
Typically, the work procedure entails a trader working with comprehensive market research and defining a potential investment strategy, which is converted into computer code by a team of programmers.
The result is a classic ‘if… then…’ kind of logic, with the algorithm being designed to act in a particular way under specific market conditions.
This could, for example, mean that the algorithm rapidly buys a stock if it spots demand for that stock is suddenly increasing – and then sells it again seconds (or microseconds) later, if the price has risen.
Even tiny gains per algorithmic transaction can be attractive if thousands of them are conducted every minute – which is what many first-generation algorithms do.
Lost USD 460 million in 45 minutes
And what do these first-generation algorithms have to do with the principal-agent problem?
In some ways, nothing. After all, the algorithm making certain investment decisions has no will of its own or independent interests to promote. Its transactions are based solely on the instructions it is given.
Yet, this form of algorithmic trading entails principal-agent problems, though of a more indirect form.
Perhaps the individuals developing the algorithms are prioritising their own interests rather than those of their company (the principal).

In fact, sociological studies of companies specialising in this form of algorithmic trading indicate that often they are set up so that their teams of algorithmic traders compete with each other.
Members of management have trouble keeping track of which algorithms their traders are developing, and how the internal systems interact with each other – for instance, they can trade with and against each other, which can be both illegal and result in losses.
It is therefore hardly surprising that it can all go horribly wrong. The worst example is the American company Knight Capital, which, back in 2012, lost USD 460 million in just 45 minutes because its system suddenly and unexpectedly activated some old algorithms.
The company ended up being acquired by a competitor.
Algorithms now have ‘a free hand’
Since the mid-2010s, an increasing number of companies have begun supplementing or entirely replacing their first-generation algorithms with a new generation of algorithms.
These second-generation algorithms are based on various forms of machine learning and are designed to develop their own investment strategies based on vast volumes of market data.
Unlike first-generation algorithms, which, as mentioned, are designed completely and utterly by humans, and where specific (teams of) individuals propose ideas for certain investment strategies, with second-generation algorithms, humans play a more limited role.
Naturally, some individuals develop the specific machine-learning architectures, and some individuals select and clean the data fed into the algorithms.
But unlike their predecessors, the whole idea of second-generation algorithms is for the algorithms themselves to work out which, when and how many shares and other financial products to buy and sell.
[How do you control what you do not understand?]
This semi-autonomy, where algorithms make real-life investment decisions, simultaneously involves a reunion with and a reconfiguration of the principal-agent problem.
In principle, second-generation algorithms can teach themselves to make profitable investments in a way that is either unethical or directly illegal – and that is definitely not in line with the intentions of the individuals who developed the basic architecture in the first place.
As with the Leeson example, this can incur considerable risk for the company (the principal).
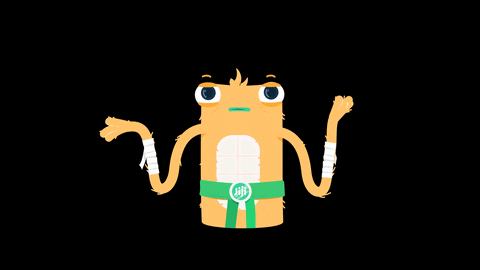
From a principal-agent viewpoint, the challenge is that, as investment decisions are now being made by algorithms, undesirable conduct cannot be regulated with conventional methods, such as elaborately detailed contracts or financial incentives.
Challenges like this have sparked suggestions that such self-learning algorithms should be wrapped in a thick ‘straight jacket’ of controls that, for instance, limit how much they can invest.
Such control mechanisms are important but fail to address the more fundamental problem characterising the most sophisticated machine-learning architectures, the so-called ‘deep neural networks’:
These can be so complex that not even the individuals who developed them can figure out why the algorithms are making specific investment decisions.
When that is the case, defining the correct control mechanisms is difficult.
Time to rethink the financial markets
This also highlights the key change in the financial markets in recent decades.
Whereas Leeson’s dubious conduct could perhaps be attributed to psychological factors and constituted a principal-agent problem that could, in principle, have been managed with an agreement between the relevant parties, second-generation algorithms cannot be understood in terms of a psychological or traditional solution based on the principal-agent theory.
Since second-generation algorithms dominate the financial markets, we must rethink how these markets are understood, analysed and regulated.
Here, psychology steps back and data science takes centre stage.
The challenging task is now to find methods that explain how, at first glance, non-transparent second-generation algorithms function – specifically how they make concrete investment decisions. One branch of data science is currently working on precisely that.
Only when the internal dynamics of neural networks can be explained down to the ground, will it be possible to understand and regulate real-life markets in a satisfactory manner.
Comments