Students build neural network that can recognize skin cancer
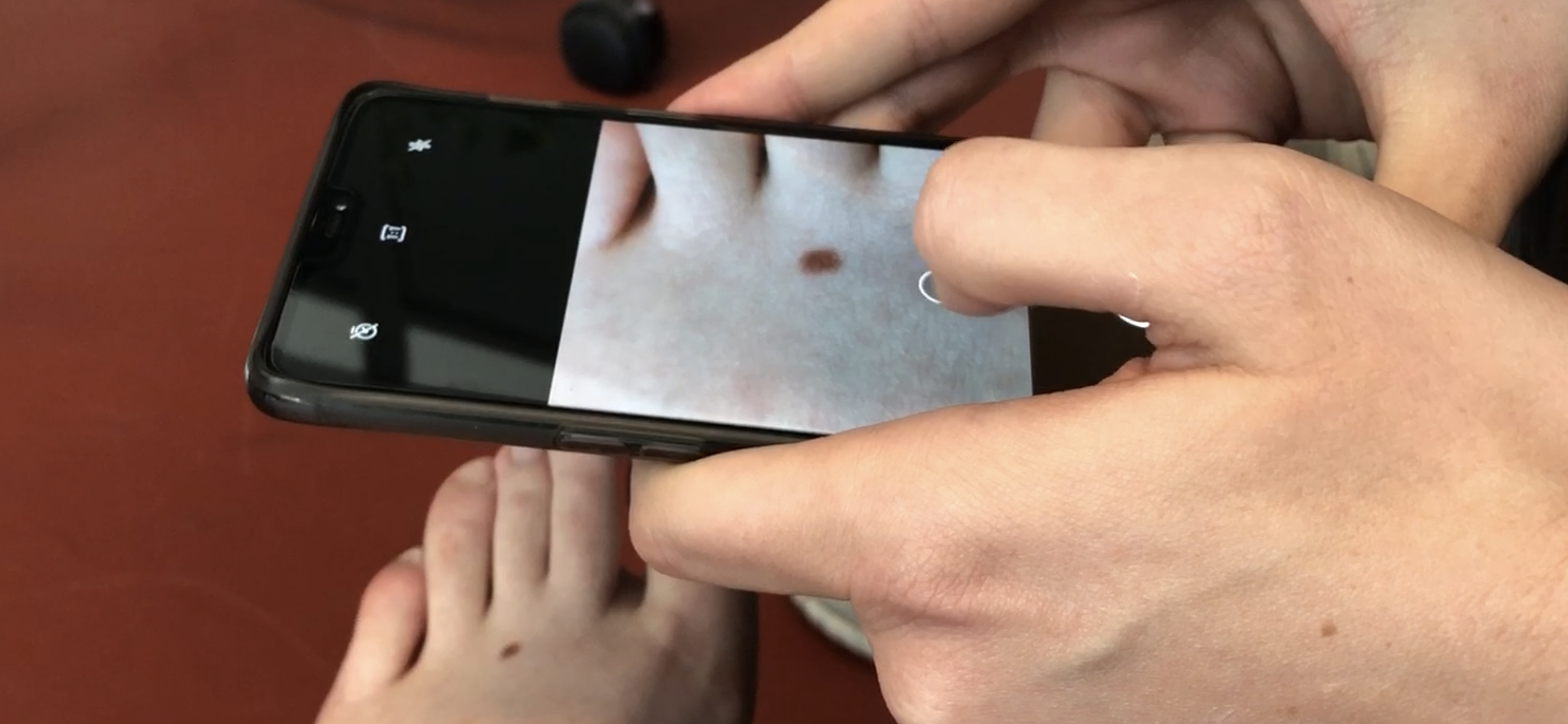
Two CBS students have made an app that can scan moles for skin cancer. (Photo: Anne M. Lykkegaard)
People have trained neural networks to recognize the differences between dogs and muffins. CBS students Mads Gade Henrichsen and Aske Bøttger have taken this one step further and built a neural network that can detect cancer with 90 percent accuracy using scanned images of moles.
“If you take a picture of a coffee pot, it’ll tell you that it’s got cancer,” says Aske Bøttger, cand.merc.it student at CBS and continues:
“So when you take a picture of a birthmark, you have to be really accurate and make sure that you don’t have other objects in the frame, like watches or socks.”
Aske Bøttger and his fellow student, Mads Gade Henrichsen, who also studies data science at the cand.merc.it, are explaining the concept of their neural network, which they’ve trained to recognize skin cancer with 90 percent accuracy.
A neural network simulates, to a certain extent, a functioning brain and can be trained for various applications. In this case, detecting abnormalities in moles that may be a precursor to cancer.
There are plenty of examples of neural networks that have been taught to tell muffins and dogs apart.
Mads Gade Henrichsen
The smart thing about neural networks, according to Mads Gade Henrichsen, is that they’re far better at remembering than humans will ever be. The two students have trained the neural network on an open source dataset with 10,000 images of moles with and without cancer, and it remembers every single one of them.
“Skin cancer is complex, as it comes in all shapes, colors and sizes. But a neural network will remember every single mole it has ever seen, and whether it had cancer or not,” says Mads Gade Henrichsen.
And this is super smart, explains Aske Bøttger.
“This kind of tech is not designed to stand alone and do the doctor’s job. And it’s certainly not supposed to hand out diagnoses, but it will tell you whether it’s a good idea to see a doctor or not. However, it can be used as a second opinion for doctors and maybe even encourage people, who don’t normally go to the doctor, to see one before it gets really bad,” he says.
Neural network up for grabs
The two students explain that you upload an image of a mole to the website, and a few seconds later, the network will tell you whether you need to get it checked by a doctor or if it looks normal.
When the neural network scans the image, it scales it down to 200 x 200 pixels and compares it to moles that it has been trained on. In total, the network uses 24 million different variables such as color, size and structure when it looks at the image to determine the result.
“The idea is that people get the opportunity to check themselves. And this might be the push they need to actually go and see a doctor if it turns out that there’s a risk of cancer,” says Asker Bøttger.
On the question of whether the test will give you a false sense of security, Mads Gade Henrichsen answers:
“Ninety percent accuracy means that the neural network is wrong 10 percent of the time. To alleviate this problem, the website will classify your mole as cancer if the picture shows a higher than 30 percent risk of cancer, even though it’s more certain that another non-cancer mole is correctly classified. This creates false positives; but in the case of cancer, it’s better to be safe than sorry,” he says.
Aske Bøttger adds:
“And even if the neural network tells you that your mole most likely doesn’t contain cancer, the website will still encourage you to see a doctor if you want to be completely sure that the mole doesn’t contain cancer.”
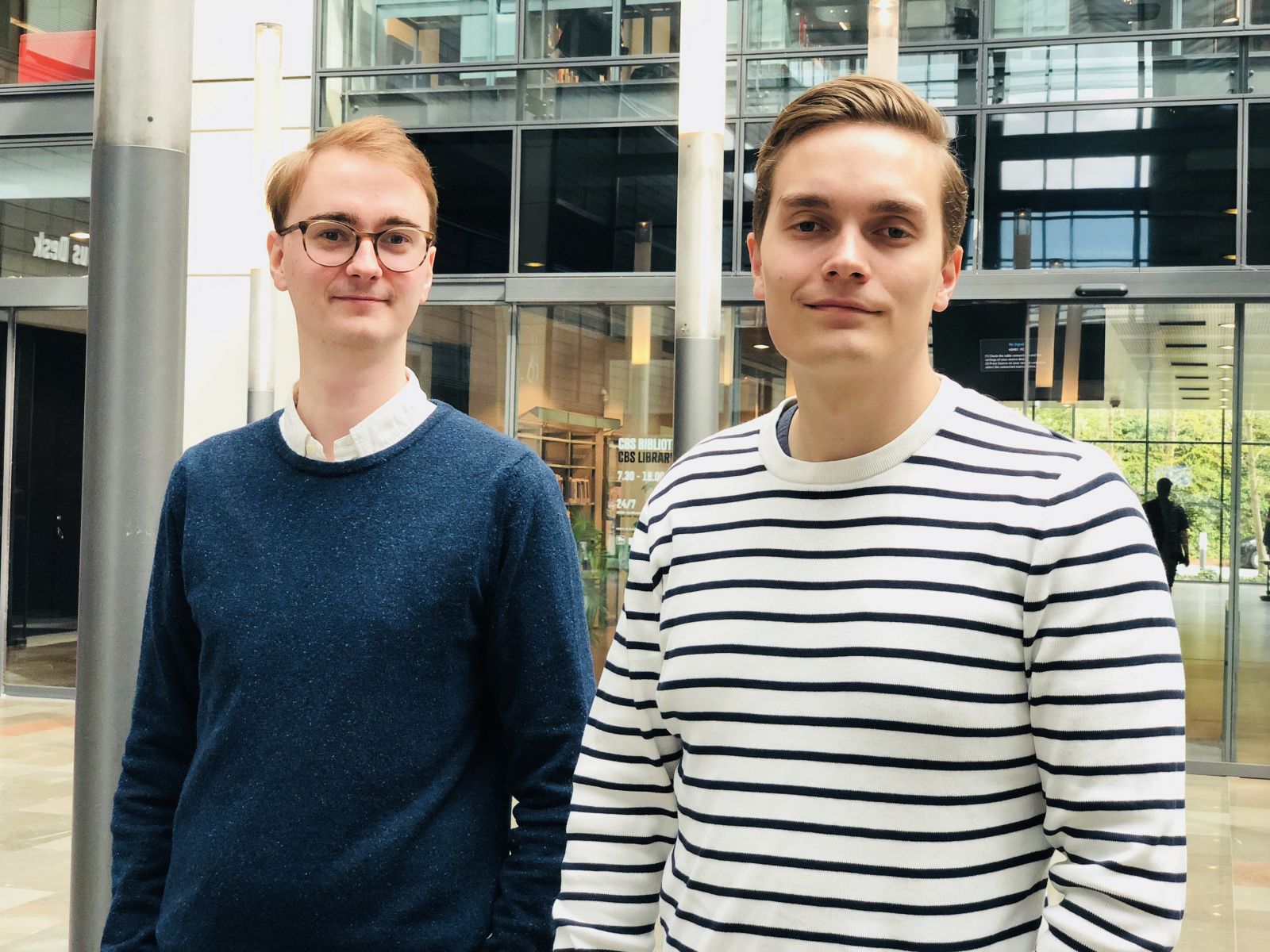
Mads Gade Henrichsen explains that the neural network is free to use, and that it might be interesting for organizations to use in, for example, awareness campaigns.
“It doesn’t cost us anything to run it. So we don’t see why we should take it down or keep it to ourselves,” he says.
Telling dogs, muffins and moles apart
Mads Gade Henrichsen got the idea for the neural network when he took classes in machine learning during his exchange. He made simple use of the concept and learned how to teach a machine to classify different images. But Mads Gade Henrichsen thought that the concept had more potential.
“There are plenty of examples of neural networks that have been taught to tell muffins and dogs apart. But I thought that it could be used for something more useful – like detecting skin cancer,” explains Mads Gade Henrichsen.
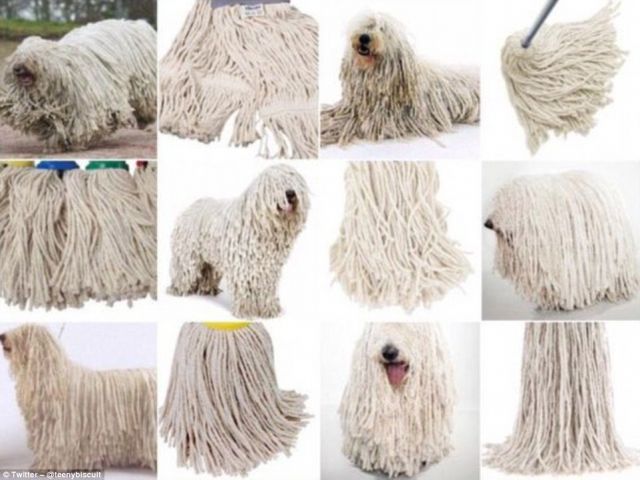
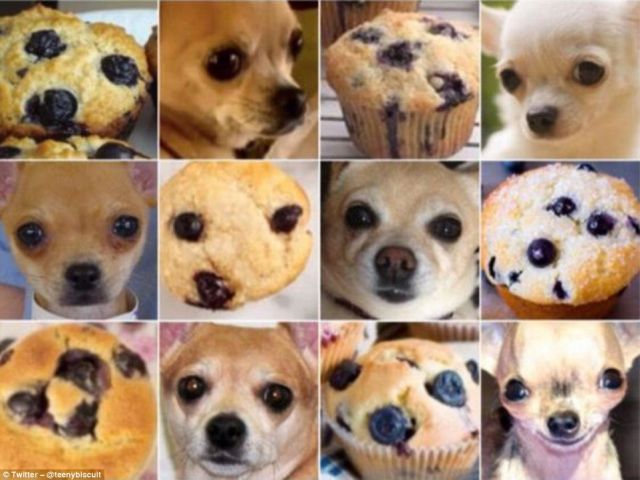
When he returned to CBS, he decided to base his bachelor project on the neural network and continued to work on the project when he started his master’s degree. That’s when Aske Bøttger joined the project and things started to evolve.
“A fellow student encouraged us to apply for the Nordic Health Hackathon in Helsinki. You get 24 hours to solve a health-related issue. When you apply for it, you get the chance to be invited with all expenses paid. So we applied for it, and got invited to the hackathon,” explains Aske Bøttger.
The two CBS students wanted to improve their neural network before the hackathon this spring, so they started to search for people who would be interested in helping them out. Luckily, Mads Gade Henrichsen’s work granted them a case to connect an external graphics card to a laptop and BitLab at CBS lent them a graphics card. This allowed even bigger calculations to be run on the neural network.
“We thought that it was just for students, but it turned out that companies such as IBM and Microsoft had employees competing too. Still, we were the only group who could present a finished and functioning solution by the end of the hackathon,” says Aske Bøttger.
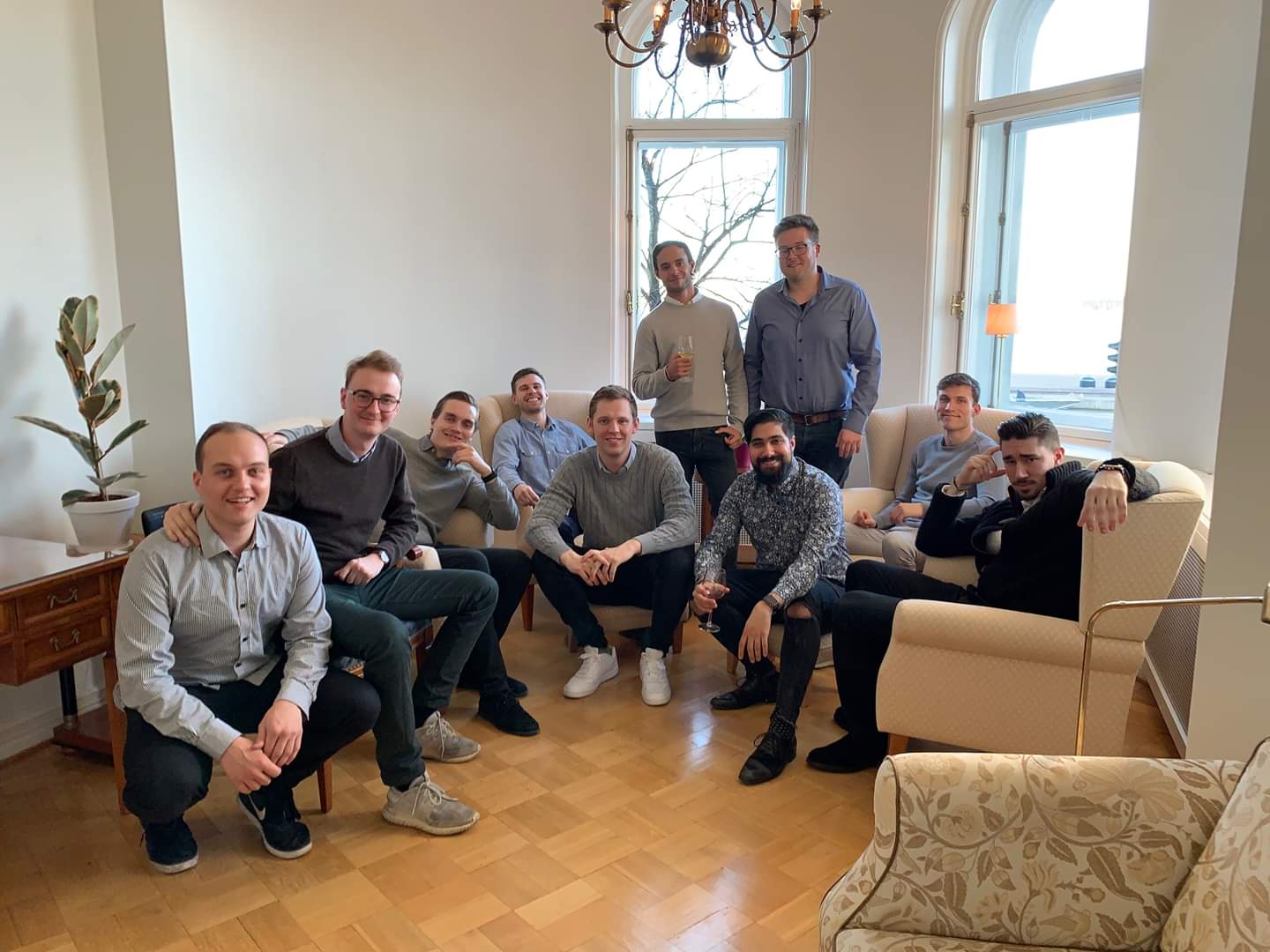
At the hackathon, they only announced the winner, so Mads Gade Henrichsen and Aske Bøttger asked the officials if they could give them an indication of their placement in the competition.
“Without it being official or anything, they told us that we won second place,” says Aske Bøttger.
Both Mads Gade Henrichsen and Aske Bøttger think they’ll continue working with machine learning at CBS, and maybe in the future as well.
“Being part of the hackathon and working with machine learning in this way has given us a clear idea of how you can use something technical and complex for everyday use. Something that’s not just interesting to us, but for other people too,” says Aske Bøttger.
Comments